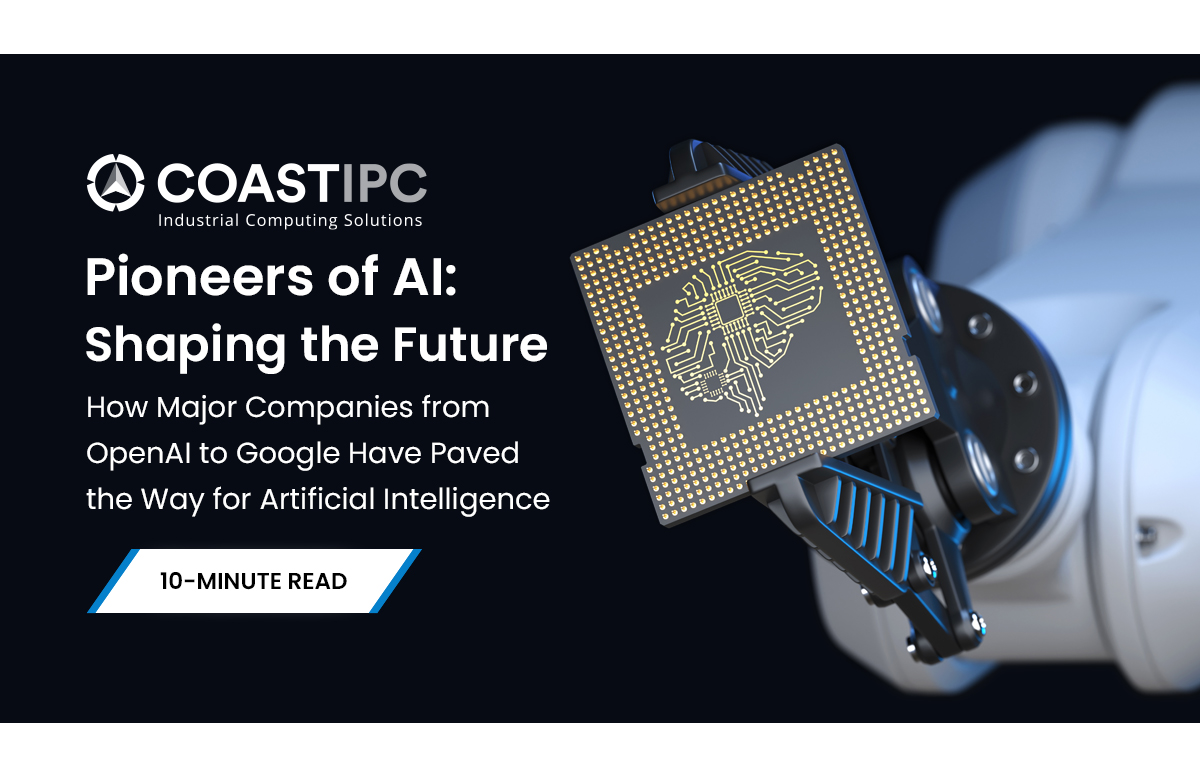
Artificial intelligence (AI) remains a hot button issue and popular topic not just in the industrial realm, but also in everyday discourse, as technologies like generative AI and large language models become more prevalent in everyday life. While the great debate around AI will persist, there is no doubt that software featuring machine learning and deep learning algorithms have added value to industrial processes from automotive manufacturing to food and beverage production, or outside the factory floor in areas like agriculture or autonomous vehicles.
While many of the AI contributions to the industrial space come from smaller niche markets such as machine vision and automation, significant advancements from industry giants have paved the way for this progress in a myriad of different ways. This article looks at the contributions from six major companies and how they have opened up new possibilities in industrial settings such as manufacturing, warehousing, and logistics, and beyond.
OpenAI
Founded in 2015 as a nonprofit AI research company by a team of experts, with Elon Musk and Sam Altman serving as initial Board members, OpenAI developed the most well-known set of large language models (LLMs) — ChatGPT — which helped bring the capabilities of LLMs and AI to the world stage. In addition, OpenAI introduced DALL-E, a deep learning model that can generate images from textual descriptions.
At first it seemed as if ChatGPT might not be much more than a sophisticated chatbot, but it quickly became controversial as it was used by students for plagiarism and by scammers for crafting believable phishing emails in multiple languages; others decried its general ability to spread misinformation or even create social unrest. The technology continues to advance, however, and OpenAI even plans to develop a watermark to identify ChatGPT-based text.
Even still, LLM technology such as ChatGPT has proved useful for some, including in the industrial automation space. Global automation company Beckhoff, for example, leverages LLMs in its TwinCAT XAE engineering environment to allow end users to quickly and easily develop a TwinCAT project, which the company says increases productivity in control programming. Siemens and Microsoft also collaborated on a project aimed toward accelerating the programming of programming logic controllers. In that project, engineers leveraged ChatGPT and other Microsoft Azure AI services to generate PLC code while also using the technology to identify errors and generate step-by-step solutions. Other companies that have leveraged or plan to leverage similar AI tools in their products include Boston Dynamics, Rockwell Automation, Doosan, and more.
OpenAI also recently announced plans to restart its robotics research group after a three-year hiatus, so the company remains an important one to monitor when it comes to following developments in the industrial automation space.
Google’s AI division, simply titled Google AI, is dedicated to advancing AI technologies. Its subsidiaries include two separate research groups: Google DeepMind and Google Brain, which merged in 2023.
In 2010, DeepMind was founded in the UK with an interdisciplinary approach to building general AI systems. DeepMind introduced neural Turing machines, a neural network architecture inspired by models of human memory and a digital computer’s design that can access external memory resources like a traditional Turing machine. DeepMind also created neural network models designed to play video games, including its AlphaGo and AlphaZero programs, the former of which made headlines when it beat professional Go player Lee Sedol. One of the company’s earlier achievements also involved gaming, as its Deep Q-Networks algorithm learned to play 49 Atari games on its own by observing raw pixels on the screen, with the instructions to maximize the score. DeepMind also introduced AlphaFold, a deep learning program that accurately predicts 3D models of protein structures, which has led to major strides in biology.
In addition, DeepMind introduced the WaveNet text-to-speech system, which provided the voice of the Google Assistant and laid the groundwork for much of the technology used in generative AI today. Google Brain was also a deep learning team under Google AI that formed in 2011 with the goal of exploring how modern AI could transform Google’s products and services. Google Brain’s research laid the foundation for the infrastructure Google runs on today, including open-source software like JAX and TensorFlow, the latter of which has become an enormously popular open-source machine learning framework, including within the industrial automation space. Additional notable achievements from Google Brain included the development of the Transformer architecture — a deep learning framework that that supports most LLMs today — as well as several more projects for image enhancement, encryption, translation (Google Translate), robotics, and more.
Looking toward the future, Google AI has the potential to further revolutionize the field of AI and its tangentially related industries and applications as they work toward their goal of “making AI helpful for everyone” — which will certainly make them an interesting watch in the years to come.
Microsoft Research
Founded in 1991 by Bill Gates, Richard Rashid, and Nathan Myhrvold, Microsoft Research set out to advance computing and solve difficult problems through technological advances. Over the years, the company has made great strides when comes to advancing AI, having filed several hundred (697 as of 2019) AI patents, with annual spending of $10 billion to $14 billion per year since 2010. As a result, Microsoft Research has added AI capabilities to many of its products over the years, including Bing, Cortana, Dynamics 365, LinkedIn, Microsoft Translator, and Windows 11, with Co-Pilot+.
Introduced in 2008, Microsoft Azure offers more than 600 total services, including service (SaaS), platform as a service (PaaS), infrastructure as a service (IaaS), data management, storage, messaging, and Internet of Things options. Specifically in the AI realm, Azure also features Azure Machine Learning Studio, which offers tools and machine learning frameworks for developers to create their own AI tools. Azure Machine Learning provides a means for machine learning professionals, data scientists, and engineers or developers to train and deploy AI models and manage machine learning operations (MLOps).
Tools and features available in Azure Machine Learning that can benefit users today include a purpose-built AI infrastructure, the ability to quickly iterate data preparation, a model catalog, a feature store, and the ability to create machine learning models for tasks such as classification or machine vision tasks, such as anomaly detection on a production line.
NVIDIA
Founded in 1993 by CEO Jensen Huang, NVIDIA has become a giant in the AI space. To get an idea of just how large NVIDIA is, for the quarter ending on April 28, 2024, the company reported record quarterly revenue of $26 billion, which represents 18% growth from the previous quarter and 262% growth year over year.
With the launch of the GeForce 256 in 1999, NVIDIA introduced the first product they referred to as a graphics processing unit (GPU), which helped spark the growth of PC gaming. GPUs consist of many processing units (or “cores”) that enable parallel computing capabilities and allow the devices to run several processes at once. At first, GPUs were well known for their ability to render 3D graphics; today, more than 200 million gamers and creators use NVIDIA GeForce GPUs. Over the years, however, GPU applications have expanded beyond the gaming realm.
The proliferation of the GPU is due in part to what has been referred to as Huang’s law, which references an observation made by Huang in 2018 that GPUs are 25 times faster than 5 years ago, whereas according to Moore’s law they would have seen only a 10x increase. As a result, these GPUs have found their way into many types of applications across disparate industries around the world. In the industrial space, for example, NVIDIA is nearly omnipresent. The company boasts that more than 40,000 companies use NVIDIA AI technology, that NVIDIA Drive powers all 30 of the top 30 autonomous vehicle data centers, and that more than 1.2 million developers use the NVIDIA Jetson platform for edge AI and robotics applications.
As industrial computing technologies like GPUs advance, they open doors to new applications while lowering the barrier of entry for others. For instance, the increasingly popular NVIDIA Jetson platform — which combines GPU, CPU, memory, power management, and high-speed interfaces — is a fast, low-power, and cost-effective alternative to traditional industrial computing platforms. Several models are available, all of which are supported by the same software stack. These devices have become widely popular for many edge AI deployments.
It goes beyond Jetson, of course. NVIDIA offers a wide range of GPUs and other computing devices targeted toward AI and deep learning applications. While AI or deep learning technologies aren’t new, their gain in both adoption and visibility in the industrial space comes at a great time, in terms of the overall development of GPUs and AI accelerating devices. In recent years, whether through causation or correlation of GPU advances, AI and deep learning software have become more prominent in machine vision and industrial automation applications, including segmentation, classification, defect and anomaly detection, and more.
IBM Research
During the final months of World War II in 1945, IBM initially founded its Watson Scientific Computing Laboratory at Columbia University to provide computing services to the allied forces, and then to advance the state of the art in scientific computing. In its early days, the Watson Lab designed and/or built several notable, historic computers. These include the SSEC (1948, considered by some to be the first true computer), NORC (1954, the first supercomputer), and the IBM 610 (1956, the first personal computer).
Over the years, the accomplishments of IBM Research have been staggering; their significant recognitions include six Nobel prizes, 10 Medals of Technology, five National Medals of Science, and six Turing awards. IBM Research notably invented the floppy disk, the hard disk drive, the magnetic stripe card, dynamic random-access memory (DRAM), the smartphone, the automated teller machine (ATM), and the Watson computer system.
While IBM has played a role in advancing AI since the 1950s the company has seen some notable achievements in the 21st century, including the development of the IBM Watson system in 2007. Four years later, the system beat the two highest-ranked “Jeopardy” players in a nationally televised two-game match. In 2013, the IBM Watson Developer Cloud was introduced to provide a development platform in the cloud for companies ranging from startups to large, established businesses. Then in 2017, IBM unified its natural language processing teams into the Watson Natural Language Processing (NLP) library, a machine learning tool that provides natural language processing functions for syntax analysis and pre-trained models for a variety of text processing tasks, according to IBM.
Five years later in 2022, IBM announced that the NLP tool plus two other IBM Watson software libraries could be embedded into apps, helping to open up AI opportunities for companies of all types. This was done, according to IBM, to allow partners, clients, and developers to more easily, quickly, and cost effectively build their own AI-powered solutions to bring to market. A year later, IBM announced watsonx, an AI and data platform that allows users to train, tune, and distribute models with generative AI and machine learning capabilities.
Of course, this is just a brief snapshot of the contributions that IBM has made to AI over the years. Given the long, storied history of the company, IBM remains a company to watch going forward for developments in the AI space.
Intel
When it comes to advances in industrial computing that have helped open new possibilities in AI, Intel is another major player. Intel was founded in 1968 and was initially well known for developing logic circuits using semiconductor devices, but over the years, the company’s list of accomplishments is vast. This includes the development of the x86 architecture, the release of the first microcontroller (Intel MCS-48), the release of the first commercially available microprocessor (Intel 4004), and the development of CHMOS (complementary high-performance metal-oxide-silicon) technology, which was integrated in the 80C49 and 80C51 microcontrollers and used less power, ran faster, and produced less heat than previous chips, according to the company.
Intel has grown into one of the largest technology companies in the world, supplying microprocessors for most computer companies and eclipsing more than $70 billion in revenue in 2018. Now in its sixth decade, Intel focuses on technologies such as AI, edge computing, and 5G wireless networking with the goal of “creating world-changing technology that improves the life of every person on the planet.” In the industrial space, Intel has helped advanced AI and deep learning technologies in several ways, including with the Intel Movidius VPU (vision processing unit).
VPUs are microprocessors that are designed specifically for image acquisition and interpretation. The devices are less powerful than GPUs but are a low-cost and low-power means for delivering computational power for machine vision and AI applications at the edge. The Intel Movidius Myriad X interfaces with a CMOS sensor to preprocess captured images, move the images through a pretrained neural network, and output results while consuming less than 1W of power. Intel also offers a range of AI accelerating processors, including Xeon scalable processors, Max processors (CPUs and GPUs), and Habana Gaudi, Gaudi 2, and Gaudi 3 accelerators.
On the software side, Intel also has a range of AI tools and platforms, including framework optimizations, AI libraries, and integrated hardware/software platforms optimized for AI. In addition, Intel has a range of AI PCs and a wide library of resources, including use cases, guides, kits, and tool selectors.
Moore’s Law refers to the observation made by Intel co-founder Gordon Moore that the number of transistors on an integrated circuit doubles every two years with minimal rise in cost. To this day, Intel uses Moore’s law as inspiration to innovate and deliver the future. As we march on into the future of AI and industrial computing, new developments from Intel will warrant our full attention.
CoastIPC
AI advancements, whether from niche companies or major conglomerates within the industrial automation space, will continue to benefit businesses around the world. From machine vision and robotics to autonomous vehicles and intelligent transportation applications, CoastIPC will help you identify the best components for your AI application.
For 15 years, CoastIPC has built the brains for the smartest machines in the world. We support the development of rugged, industrial PCs and machines through collaboration, hardware selection, and customization, building products with a quality process second to none. This includes industrial PCs, GPU and VPU accelerated computing products, NVIDIA Jetson products, and much more. At CoastIPC, we live at the edge of industrial computing performance. Our experts are ready to discuss AI, deep learning, machine learning, industrial computing advancements, or any other technology, old or new. Contact us anytime via email, phone, or chat. Check out our blog or follow us on LinkedIn to stay up to date.